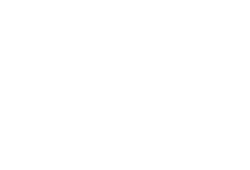
Evolving the Workforce for A.I. Adoption
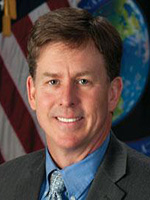
Guest blogger: Doug McGovern, Chief Technology Officer for Intelligence Programs, IBM Global Business Services
This panel was part of a Machine Learning and Artificial Intelligence (A.I.) workshop held at the National Geospatial Intelligence Agency’s (NGA) headquarters in Springfield Virginia and was attended by industry, academia and Government. Keynotes were provided by several Government Senior Executives from the Intelligence Community.
There were three other panels during the workshop that expounded on the virtues of A.I., the hard problems that Machine Learning and A.I. could solve, the imperatives for adopting A.I. solutions and challenges in learning to trust A.I., but my panel was focused on the human side of the equation and what we need to do to prepare ourselves and the workforce. Panel members included the Director for Analytical Tradecraft from NGA, the Professor of Geospatial Intelligence Practice from Penn State University, the Director of the Center for Geospatial Intelligence at the University of Missouri and the Vice President for Professional Development at the U.S. Geospatial Intelligence Foundation. We explored the challenges in today’s approaches and practices around training and education that don’t necessarily match the demands for excelling in this fast-moving technology space. We discussed the lagging federal government investment in A.I. research and development in the U.S. compared with other countries and the challenges of retooling segments of the workforce as technology adoption changes the nature of traditionally human roles and functions in the workplace. Some of the interesting topics and concepts we uncovered are shown below:
- We’ve seen many of these workforce issues over the last century as technology has been adopted to augment and often times replace the human in repetitive or dangerous functions…is it different with Machine Learning and A.I.?
- Does history present any examples of successful re-educating, re-training and re-tooling the workforce whose jobs have been displaced by machines?
- How much does the operator/user need to know about what’s “under the hood” to have confidence that data ingest, A.I. models and algorithms are functioning properly? Does everyone have to be able to understand the detailed coding, logic and training history underlying the A.I. to trust the outputs?
- How will we integrate “A.I.s” into operations? Will we bring them right out of the “factory” (like we hire students from universities with minimal experience) and give them assignments of growing responsibility and training or will we hold them off and keep them in training until they can perform like seasoned experts?
We recognized that most of the conversation around Machine Learning and A.I. today is centered around technology aspects and that we are fairly early in the conversation about the workforce implications. I welcome you to join the conversation!