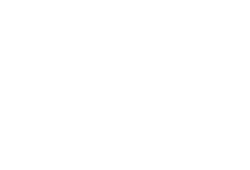
The Key to Modern Governmental Supply Chain Practice
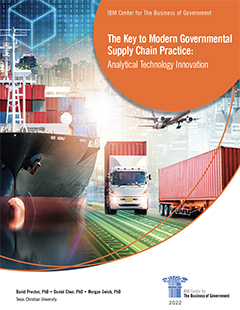
Over the past few years the COVID-19 pandemic has done much to expose and highlight critical gaps and flaws in the global supply chain. While demand for products and services continues to surge, key players in the supply chain delivery value chain struggle to meet that demand in a timely fashion. Scarce supply has resulted in increasing prices for finished goods and raw materials and an inflationary global economy.
With this scenario as context, recognize that the United States government, including federal, state, and local entities are estimated to have purchases that contributed about $3.18 trillion, or about 17 percent of the total GDP in 2018. In addition, the cost of operating supply chains is estimated to contribute up to two thirds of the final cost of goods sold. Any improvement in supply chain operations achieved through effective supply chain management (SCM) will have a significant positive impact on timely delivery and appropriate pricing of goods and services. Many of these improvements can be realized through analytical or technology improvements, yet both commercial and government entities have not tapped into the full potential of technologies and analytical approaches available to them.
To address this critical issue, the IBM Center for the Business of Government commissioned a report researched and produced by Dr. David Preston and his colleagues Dr. Daniel Chen and Dr. Morgan Swink of Texas Christian University. The report, “The Key to Modern Governmental Supply Chain Practice: Analytical Technology Innovation,” provides insight into the impact of analytics, block chain and artificial intelligence (AI) on SCM practices. The report also provides the reader with three frameworks that are helpful for designing and implementing an effective supply chain practice, enabled by technology and analytics. The first framework examines the key drivers of analytics usage for SC practice, the second examines the influence of analytics usage on SC performance outcomes and the final framework goes deeper into the ways to optimize analytics and incorporating continuous learning for the organization.
The benefits of analytics and data-driven decision making are clear in many disciplines, but SCM lends itself to dramatically benefiting from the infusion of technology approaches for several reasons. First, supply chain operations generally cross organizational and geographical boundaries and tend to be complex. Managers of each node in the supply chain network may only have clear visibility into their own part of the delivery value chain. This often results in the tendency of SC managers to optimize the operation of their node at the expense of the entire ecosystem. However, if we use appropriate analytics to harness the complete set of operational data across the entire ecosystem, curate that data and gain actionable insights at an enterprise level, and provide that view to all SC managers, we can change behavior for the long-term benefit of the entire end-to-end supply chain.
If SC managers are armed with real-time situational awareness of the status of their supply chain, they can test scenarios that could improve operational delivery and use that learning to make continuous improvements to their operational processes. All of this can be accomplished through automation and effective use of analytics, freeing the SC managers to leverage the results and spend their valuable time making informed strategic decisions and deciding how best to invest in future transformation opportunities.
Once organizations have a handle on the basics of leveraging data and analytics to their advantage, then they can begin to add in other technologies that can inform and improve operations at the next order of magnitude. Offerings such as blockchain and AI are complementary technologies and very applicable to SC practice. As stated in the report, blockchain has the “potential to efficiently connect stakeholders of government supply chains and also monitor and document the transitions of goods across the supply chain.” Further exploration of this blockchain potential is warranted. In addition, AI can play a very important role as a part of a SC analytics portfolio of tools. As stated in the report, “machine learning and AI applications are helpful with estimating resource management related inputs and parameters to help to prepare for anomalies” that impact SC performance.
In addition, another specific category of goods and services that needs to be addressed explicitly is technology itself-- this is a daunting task, but performing cyber assessments of product sources that are part of the technology supply chain can reduce cyber risk and improve quality.
In conclusion, this report provides meticulously researched recommendations that illuminate “where to begin and how to succeed” in implementing automated SC approaches. We hope that this report will provide a view into the SCM improvement opportunities provided through practical application of analytics and emerging technologies to supply chain practice.